Boaz Barak
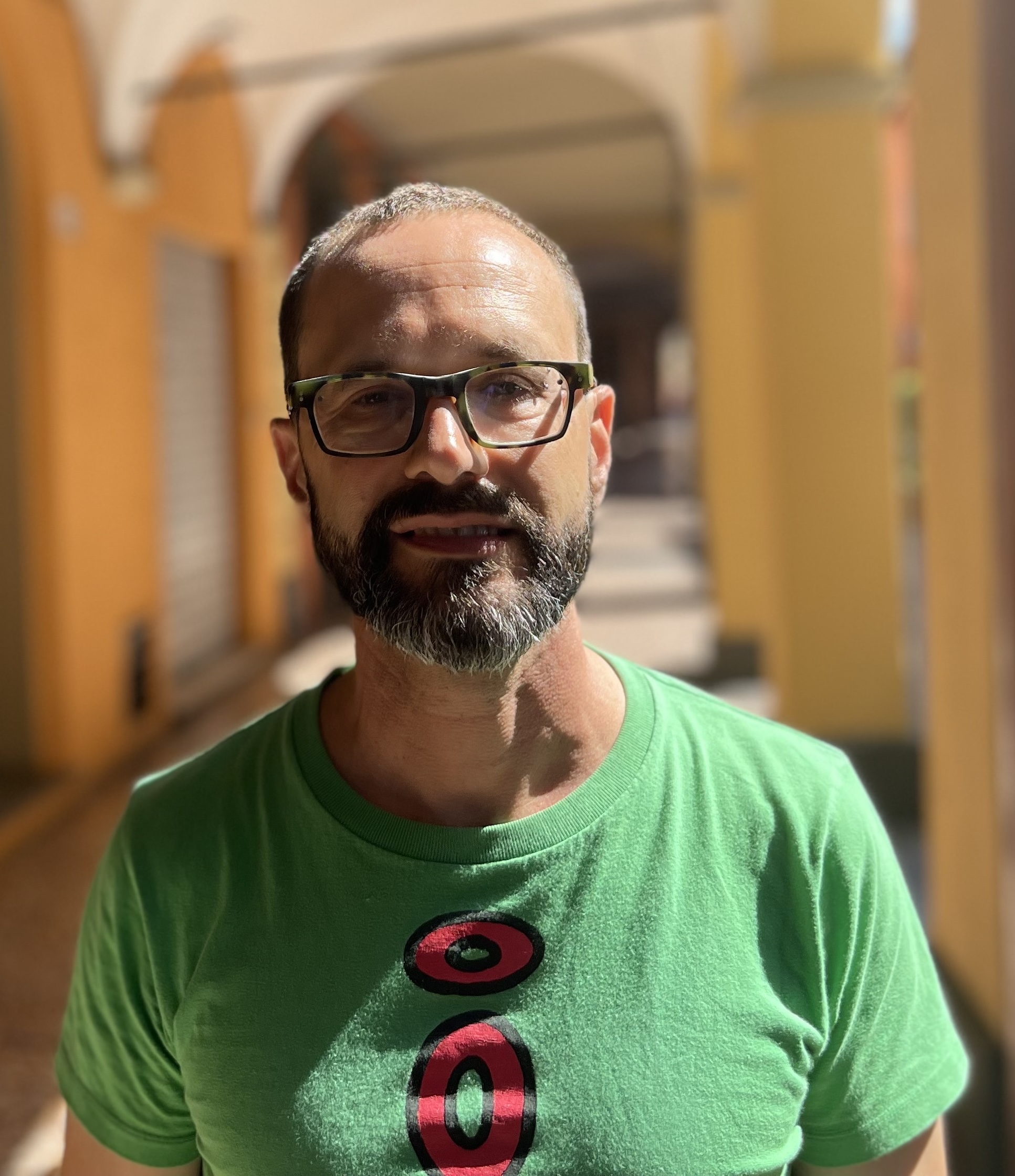
Boaz Barak (Harvard University)
Title: AI safety via Inference-time compute
Abstract: Ensuring AI models reliably follow human intent, even in situations outside their training distribution, is a challenging problem. In this talk, we will discuss how spending more computation at inference time can be used to improve robust adherence to human-specified policies, specifically using reasoning AI models such as OpenAI’s o1-preview, o1-mini, and o1. In particular I will present Deliberative Alignment: A new safety training paradigm that directly teaches the model safety specifications and trains it to explicitly recall and accurately reason over the specifications before answering. Deliberative alignment simultaneously increases robustness to jailbreaks while decreasing over-refusal rates, as well as improving out-of-distribution generalization.
Bio: Boaz Barak is the Gordon McKay professor of Computer Science at Harvard University’s John A. Paulson School of Engineering and Applied Sciences. Previously, he was a principal researcher at Microsoft Research New England, and before that an associate professor (with tenure) at Princeton University. Barak’s research interests include theoretical computer science and the foundations of machine learning. Barak has won numerous awards and fellowships, including the ACM dissertation award, Foreign Policy magazine’s list of 100 leading global thinkers, Packard fellowship, and Sloan fellowship. He was also chosen to be a Simons investigator and a fellow of the ACM. Barak is a member of the scientific advisory boards for Quanta Magazine and the Simons Institute for the Theory of Computing. He is also a board member of AddisCoder, a non-profit organization for teaching algorithms and coding to high-school students in Ethiopia and Jamaica. Barak wrote with Sanjeev Arora the textbook “Computational Complexity: A Modern Approach”.
Massimiliano Pontil
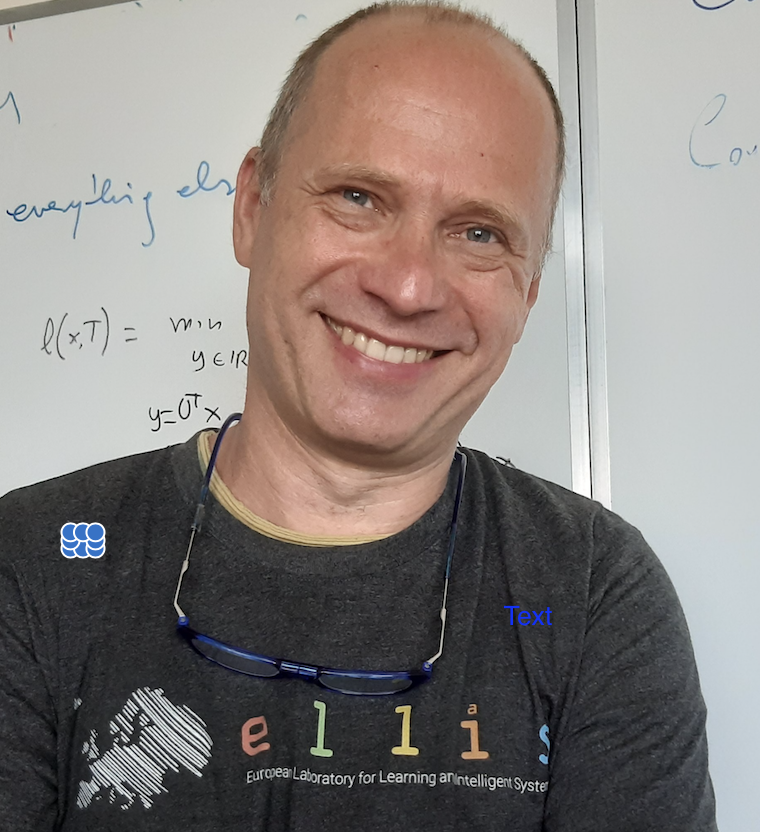
Massimiliano Pontil (Italian Institute of Technology and University College London)
Title: Linear Operators Learning for Dynamical Systems
Abstract: This talk presents a framework to learn dynamical systems through the lens of linear operators, in particular the semi-group of transfer operators and their infinitesimal generator. We review recent operator estimators, whose domain is either a reproducing kernel Hilbert space or the span of finitely many basis functions learned by a neural network. An important focus is to use the operators for forecasting future states of the system and explain system dynamics via the operators’ spectra. We present statistical learning bounds for the operator estimators and their spectral decomposition, highlighting the important role of the learned representation and the estimator’s rank. Applications to molecular dynamics and other dynamical systems will be discussed during the talk.
Bio: Massimiliano Pontil is Senior Researcher at the Italian Institute of Technology, where he leads the CSML research unit, and co-director of ELLIS unit Genoa. He is also Professor at University College London and member of the UCL Centre for Artificial Intelligence. He has been active in machine learning for over twenty-five years, working on theory and algorithms, including the areas of kernel methods, learning dynamical systems, meta-learning, multitask and transfer learning, sparse estimation, and statistical learning theory. More information on Massimiliano’s research interests and accomplishments can be found here: https://www.iit.it/people-details/-/people/massimiliano-pontil.
Claire Vernade
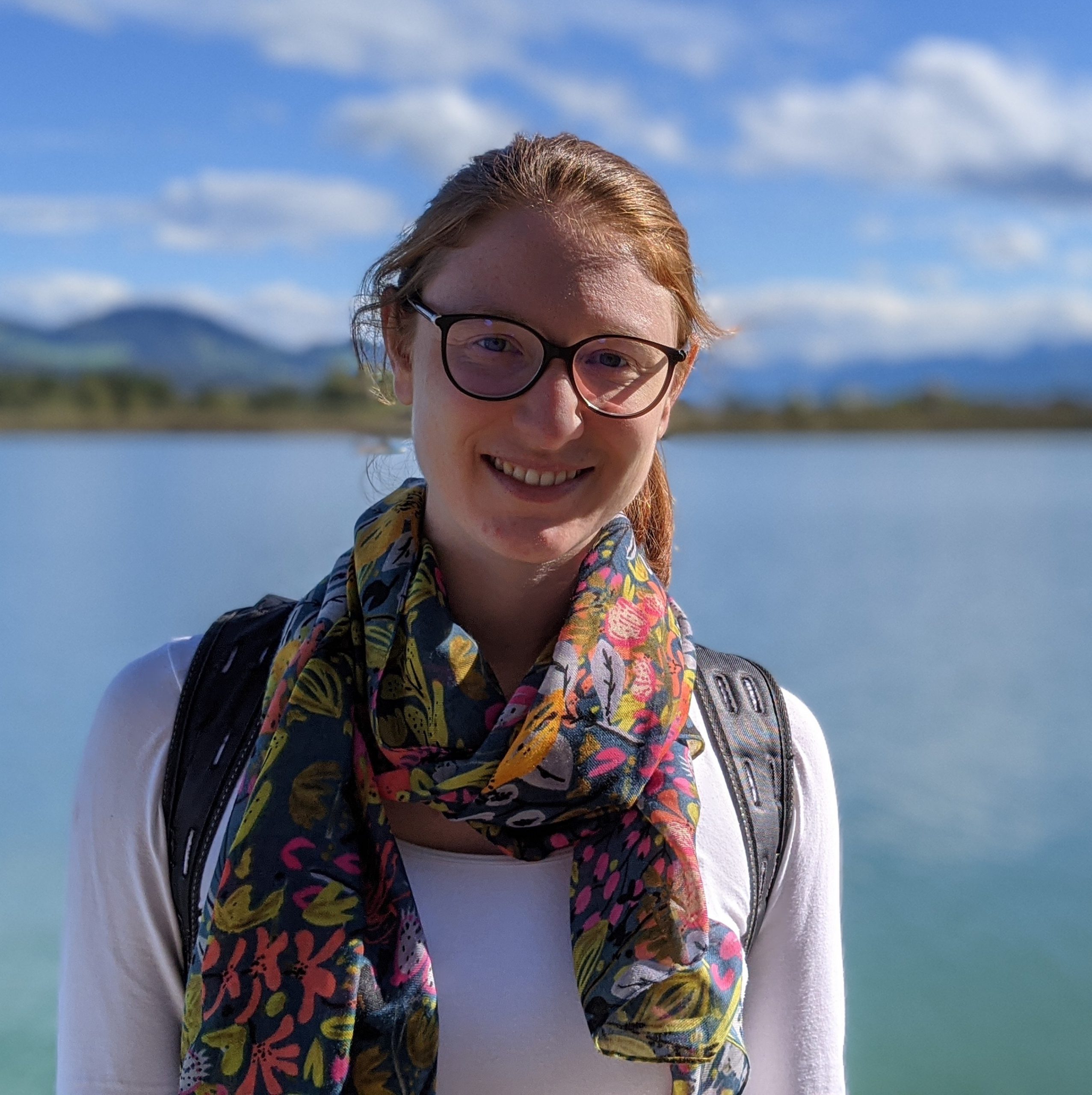
Claire Vernade (University of Tübingen)
Title: RL beyond expectations: Planning for utility functions
Bio: Claire is a “Group Leader” (equivalent Assistant Prof) at the University of Tübingen, in the Cluster of Excellence Machine Learning for Science. She was awarded an Emmy Noether award under the AI Initiative call in 2022. Her research is on sequential decision making. It mostly spans bandit problems, and theoretical Reinforcement Learning, but her research interests extend to Learning Theory and principled learning algorithms. Her goal is to contribute to the understanding and development of interactive and adaptive learning systems. Between November 2018 and December 2022, she was a Research Scientist at DeepMind in London UK in the Foundations team lead by Prof. Csaba Szepesvari. She did a post-doc in 2018 with Prof. Alexandra Carpentier at the University of Magdeburg in Germany while working part-time as an Applied Scientist at Amazon in Berlin. She received her PhD from Telecom ParisTech in October 2017, under the guidance of Prof. Olivier Cappé.
Nikita Zhivotovskiy
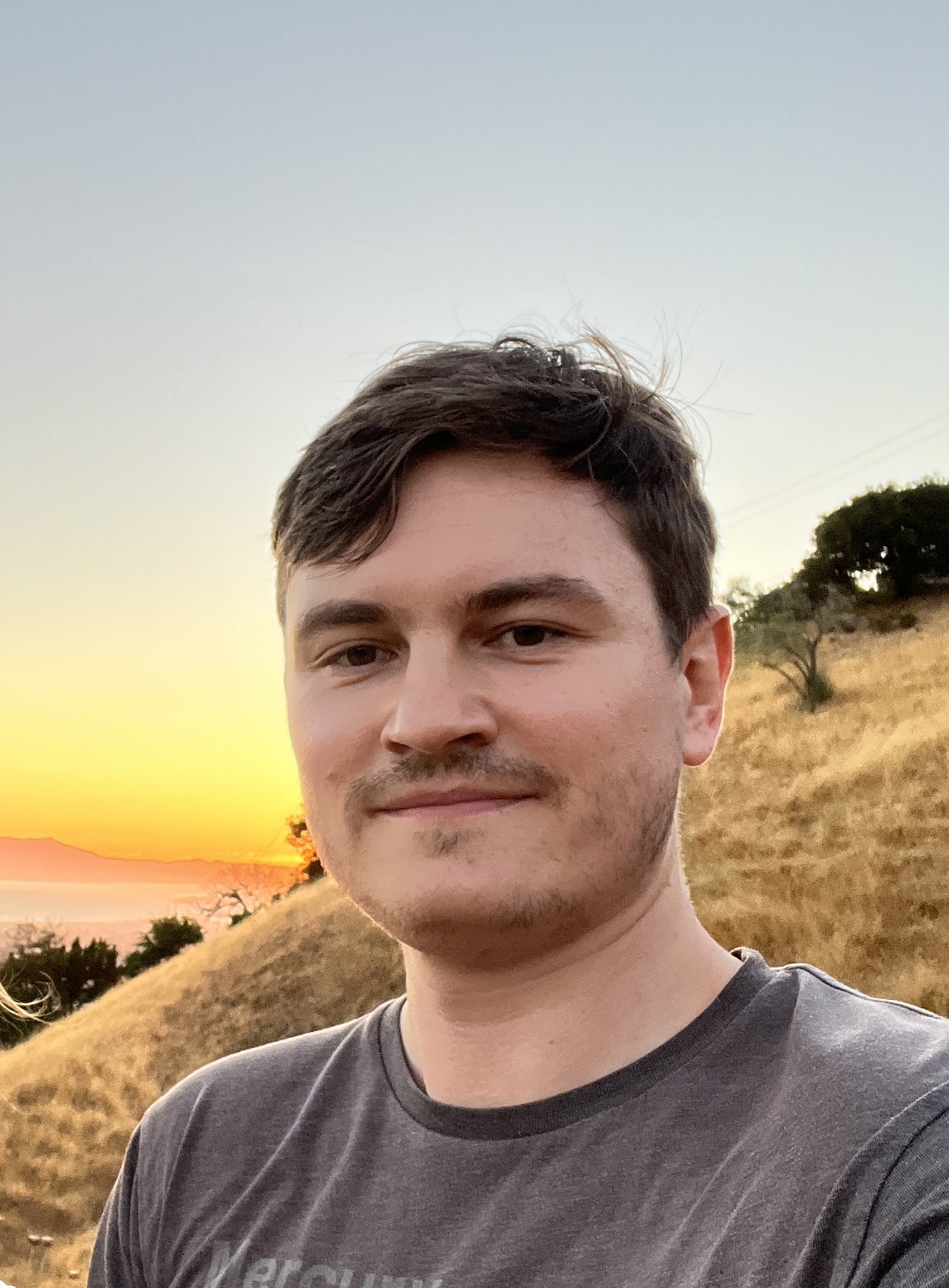
Nikita Zhivotovskiy (UC Berkeley)
Title: From Estimation to Prediction: What Assumptions Do We Need?
Abstract: Statistical Learning theory focuses on obtaining finite-sample guarantees that work for essentially all distributions of the data. A great example is the distribution-free bounds of Vapnik-Chervonenkis, which provide non-trivial classification error bounds without making assumptions on the distribution of the data apart from i.i.d. How general is this phenomenon, and to what other estimation and prediction problems and loss functions can we generalize this observation? We discuss some recent results in this direction. We begin with basic questions of estimation, such as matrix estimation, and demonstrate that in high dimensions, certain objects like sample covariance matrices can be estimated optimally under minimal moment assumptions. While moment assumptions are crucial for estimation, we further explore how these assumptions can be entirely dropped in various prediction problems, such as linear regression and logistic regression. Moreover, we highlight how logistic and square losses, rather than the binary loss, open the door to computational efficiency that was not achievable with the Vapnik-Chervonenkis results, while still maintaining the remarkable distribution-free properties of these bounds.
Bio: Nikita Zhivotovskiy is an Assistant Professor in the Department of Statistics at the University of California Berkeley. He previously held postdoctoral positions at ETH Zürich in the department of mathematics hosted by Afonso Bandeira, and at Google Research, Zürich hosted by Olivier Bousquet. He also spent time at the Technion I.I.T. mathematics department hosted by Shahar Mendelson. Nikita completed his thesis at Moscow Institute of Physics and Technology under the guidance of Vladimir Spokoiny and Konstantin Vorontsov.