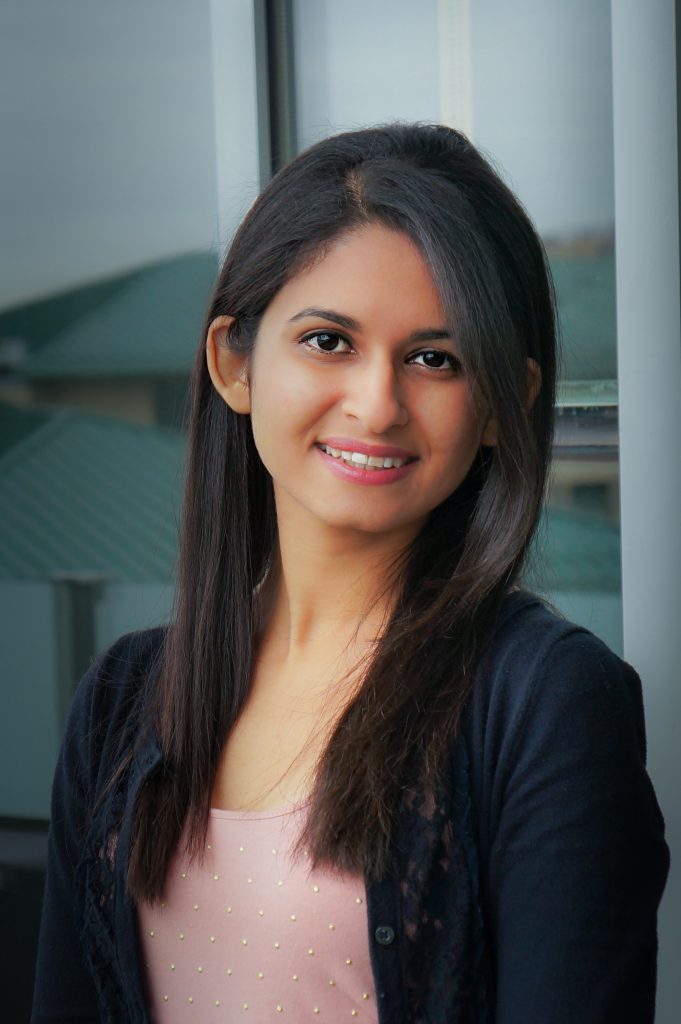
Nika Haghtalab
Talk title: Multi-objective learning: A unifying framework for robustness, fairness, and collaboration
Abstract: Social and real-world considerations such as robustness, fairness, social welfare, and multi-agent tradeoffs have given rise to multi-objective learning paradigms. In recent years, these paradigms have been studied by several disconnected communities and under different names, including collaborative learning, distributional robustness, group fairness, and fair federated learning. In this talk, I will highlight the importance of multi-objective learning paradigms in general, introduce technical tools for addressing them from a simple unifying perspective, and discuss how these problems relate to classical and modern consideration in data-driven processes.
Bio: Nika Haghtalab is an Assistant Professor in the Department of Electrical Engineering and Computer Sciences at UC Berkeley. She works broadly on the theoretical aspects of machine learning and algorithmic economics. Prof. Haghtalab’s work builds theoretical foundations for ensuring both the performance of learning algorithms in presence of everyday economic forces and the integrity of social and economic forces that are born out of the use of machine learning systems. Previously, Prof. Haghtalab was an Assistant Professor in the CS department of Cornell University, in 2019-2020. She received her Ph.D. from the Computer Science Department of Carnegie Mellon University. She is a co-founder of Learning Theory Alliance (LeT-All). Among her honors are the CMU School of Computer Science Dissertation Award, SIGecom Dissertation Honorable Mention, and NeurIPS outstanding paper award.
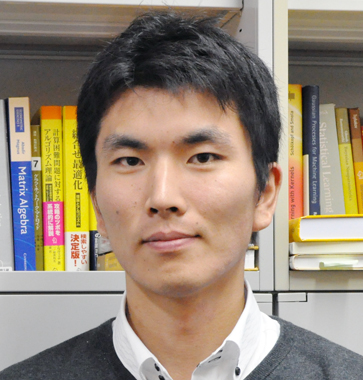
Taiji Suzuki
Talk title: Deep learning theory of mean field feature learning
Abstract: In this talk, I will show recent results of deep learning theory especially from the view point of feature learning in the mean field regime. Feature learning realizes flexible function representation ability that yields improved predictive performances especially in high dimensional settings. In particular, I deal with the mean field regime and discuss how gradient descent methods attain feature learning and how it affects the predictive performances. For example, a few step gradient descent with large step size can get out of the neural tangent kernel regime and achieve better performance than fixed-feature methods. As an optimization method, I discuss mean field Langevin dynamics in which a neural network provably achieves the global optimal solution attaining features with a better alignment. A convergence rate analysis of its fully discretized algorithm is given.
Bio: Taiji Suzuki is currently an Associate Professor in the Department of Mathematical Informatics at the University of Tokyo. He also serves as the team leader of “Deep learning theory” team in AIP-RIKEN. He received his Ph.D. degree in information science and technology from the University of Tokyo in 2009. He worked as an assistant professor in the department of mathematical informatics, the University of Tokyo between 2009 and 2013, and then he was an associate professor in the department of mathematical and computing science, Tokyo Institute of Technology between 2013 and 2017. He has a broad research interest in statistical learning theory on deep learning, kernel methods and sparse estimation, and stochastic optimization for large-scale machine learning problems. He served as area chairs of premier conferences such as NeurIPS, ICML, ICLR, AISTATS and a program chair of ACML. He received the Outstanding Paper Award at ICLR2021, the MEXT Young Scientists’ Prize, and Outstanding Achievement Award in 2017 from the Japan Statistical Society.
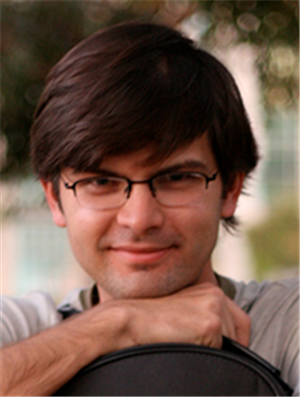
Matus Telgarsky
Talk title: “Open problems in the the approximation, generalization, and optimization of deep networks”
Abstract: This talk will present recent work and survey open problems in these three core areas of deep learning.
To start, it will review ancient (eight year old) approximation-theoretic perspectives on when depth can help, and highlight open problems in modern architectures such as transformers.
Secondly, it will review some multi-layer generalization bounds, where recent progress has encountered numerous roadblocks.
Lastly, optimization and specifically implicit bias will be discussed, where a key open problem is to identify the feature-learning abilities of modern networks.
Bio: Matus Telgarsky is an assistant professor at the University of Illinois, Urbana-Champaign, specializing in deep learning theory. He was fortunate to receive a PhD at UCSD under Sanjoy Dasgupta. Other highlights include: co-founding, in 2017, the Midwest ML Symposium (MMLS) with Po-Ling Loh; receiving a 2018 NSF CAREER award; and organizing two Simons Institute programs, one on deep learning theory (summer 2019), and one on generalization (fall 2024).
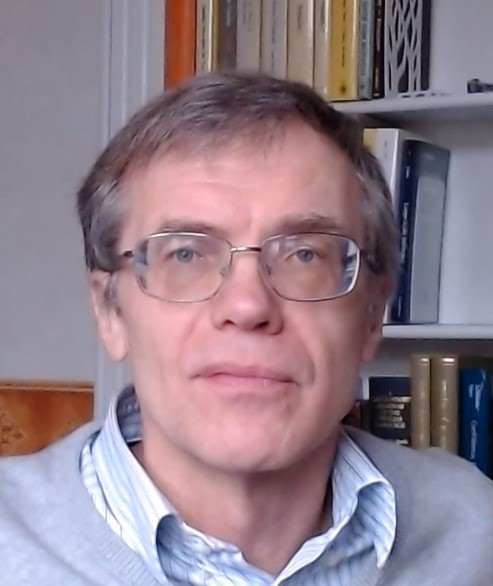
Vladimir Vovk
Talk Title: “Conformal prediction in online compression models: Twenty years later”
Abstract: My plan is to review the current state of conformal prediction in online compression models. This is the topic that I started in my ALT 2003 paper, whose expanded version was published in the ALT 2003 Special Issue of Theoretical Computer Science in 2006. Perhaps the most popular online compression model is the exchangeability model, which is standard in mainstream machine learning, but I will describe several other useful models of this kind. Online compression models are a perfect home for conformal prediction, which is a way to produce set predictions and probabilistic predictions with guaranteed properties of validity (namely, a guaranteed probability of error for set predictions and probabilistic calibration for probabilistic predictions). The properties of validity make it possible to “invert” conformal prediction to obtain online methods of testing online compression models. A recent book-length review of conformal prediction and testing is “Algorithmic Learning in a Random World” (second edition) by Vovk, Gammerman, and Shafer published by Springer in December 2022; in this talk I will give a few highlights.
Bio: Vladimir Vovk is Professor of Computer Science at Royal Holloway, University of London. His research interests include machine learning and the foundations of probability and statistics. He was one of the founders of prediction with expert advice, an area of machine learning avoiding making any statistical assumptions about the data. In 2001 he and Glenn Shafer published a book (“Probability and Finance: It’s Only a Game”) on new game-theoretic foundations of probability; the sequel (“Game-theoretic Foundations for Probability and Finance”) appeared in 2019. His second book (“Algorithmic Learning in a Random World”, 2005), co-authored with Alex Gammerman and Glenn Shafer, is the first monograph on conformal prediction, method of machine learning that provides provably valid measures of confidence for their predictions; an expanded and updated second edition has just been published (December 2022). His current research centres on applications of game-theoretic probability to statistics and machine learning.